Data-driven decision-making, artificial intelligence (AI) is a transformative force capable of revolutionizing industries. However, many organizations fall into the trap of assuming that traditional data management practices are sufficient for AI. This misconception can significantly hinder the success of AI initiatives. For data and analytics (D&A) leaders, understanding and implementing the principles of AI-ready data is crucial. This blog delves into the concept of AI-ready data, explores its defining characteristics, and outlines the steps necessary to achieve and maintain this readiness.
Understanding AI-Ready Data
The Contextual Nature of AI-Ready Data
AI-ready data is defined by its suitability for specific AI use cases. Unlike traditional data management, which often prioritizes clean and error-free data, AI-ready data must encompass the full range of real-world conditions, including errors, outliers, and unexpected values. This diversity is essential for training AI models to handle the complexities and unpredictability of real-life scenarios. The contextual nature of AI-ready data means that its fitness can only be assessed relative to the specific AI technique and application in question.
Misconceptions About AI-Ready Data
Several misconceptions can hinder the effective preparation of AI-ready data. First, there's the belief that high-quality data, as judged by traditional data quality (DQ) standards, is automatically AI-ready. AI models require data that includes anomalies and diverse scenarios to learn effectively. Second, while responsible data governance is crucial, the principles may differ for AI. AI models can benefit from data that might not fit traditional governance standards but is still valid and representative. Lastly, the idea that data can be made AI-ready in a generic sense is flawed. Data readiness depends on the specific AI technique and use case; what works for one application may not be suitable for another.
Steps to Achieve AI-Ready Data
Achieving AI readiness involves a continuous process of aligning, qualifying, and governing data according to the needs of specific AI use cases.
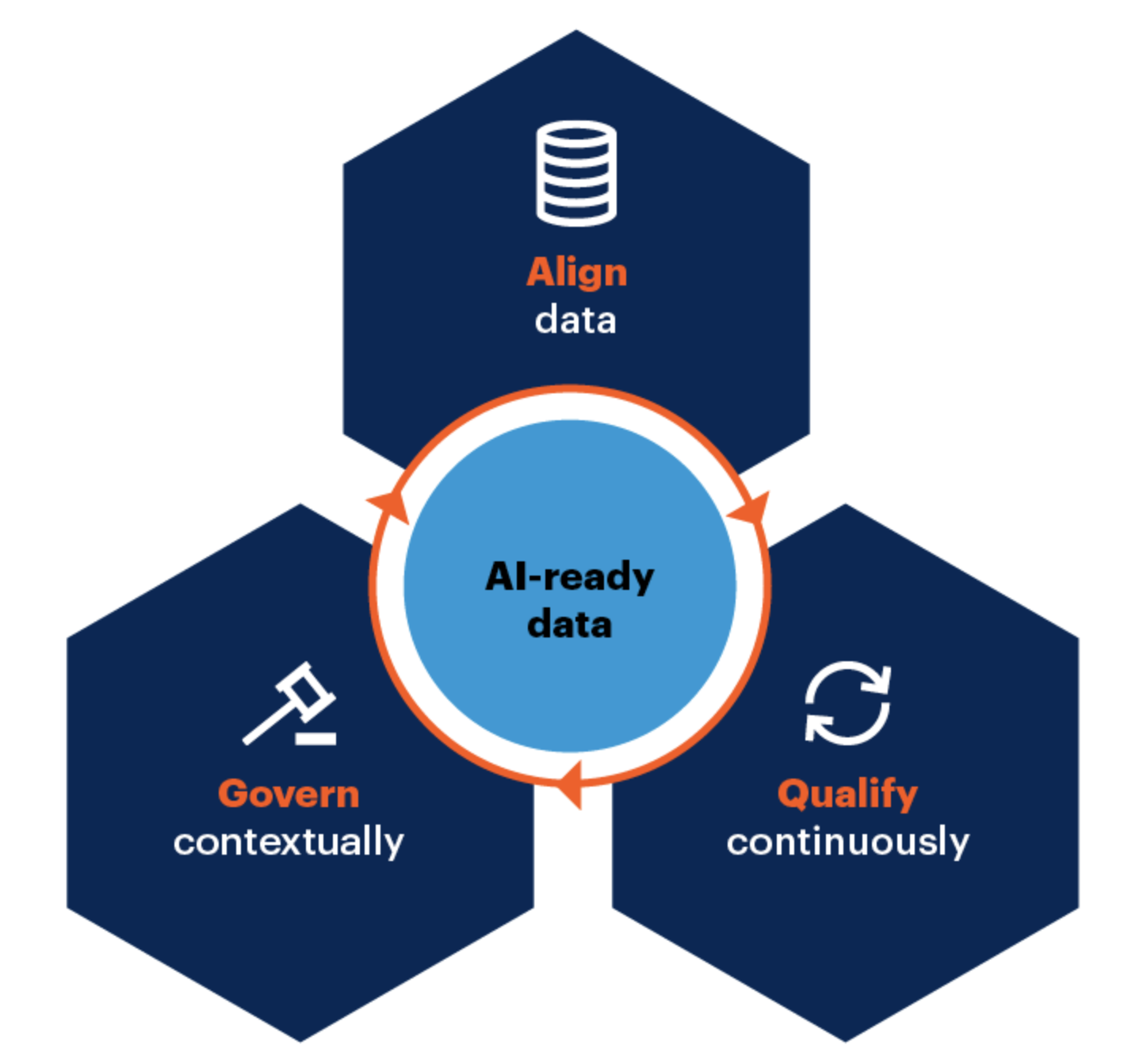
1. Aligning Data to Use-Case Requirements
Every AI use case has unique data requirements that may evolve over time. Aligning data involves ensuring it meets these specific needs. First, different AI techniques, such as generative AI or simulation models, impose unique data requirements. Understanding these nuances is critical for data alignment. For instance, training data for a generative AI model will differ significantly from that needed for a predictive maintenance algorithm. Each technique dictates specific data characteristics, which must be identified and met.
In addition to understanding AI techniques, quantification of data is essential. Ensuring there is sufficient data volume, especially for use cases with seasonal patterns, is crucial. Synthetic data can augment real data to meet these needs, providing a broader and more comprehensive dataset for training AI models. Moreover, enriching data with semantics, annotations, and labeling enhances model accuracy. For example, annotated images or videos, along with well-defined taxonomies and ontologies, can significantly improve the performance of AI models, particularly in applications involving complex visual data.
Quality of data is another critical aspect. Data must meet the quality standards specific to the AI use case, considering factors such as sparsity and completeness. High-quality data for AI is not just about being clean but also about being representative of all possible scenarios the AI model might encounter. Trust in data sources and the reliability of contributing pipelines are also paramount. Ensuring the integrity and trustworthiness of data sources helps maintain confidence in the AI model’s outputs.
Diversity in data sources prevents bias and enhances model fairness. Ensuring that the data includes a wide range of scenarios and demographics helps build fair and unbiased models. Lastly, lineage provides transparency about data origins and transformations. Understanding the data's journey from its source through various transformations and into the AI model is essential for traceability and accountability.
2. Qualifying Data to Meet AI Confidence Requirements
Qualifying data involves continuously meeting the confidence requirements for AI use cases through various parameters. Validation and verification are essential processes that ensure all data requirements are consistently met during development and operational phases. Regular checks and balances are necessary to maintain data integrity and suitability for AI models.
Performance, cost, and nonfunctional requirements must also be addressed. Data must fulfill operational service level agreements (SLAs), including response time, availability, and cost-efficiency. For instance, the cost of inference in AI applications can be significant, and data management strategies must account for this to ensure sustainable operations.
Versioning is critical for maintaining consistency and enabling rollbacks when necessary. Tracking and managing data versions ensures that any changes in data can be traced back and previous versions can be restored if needed. This is particularly important in AI, where models and data pipelines frequently evolve. Continuous regression testing helps detect issues and ensures the AI model remains reliable over time. By developing various test cases, teams can identify potential data drift and other anomalies affecting model performance.
Observability metrics and monitoring are vital for maintaining the health of AI systems. These metrics track various aspects, such as data accuracy, delivery timeliness, and operational costs. By continuously monitoring these factors, teams can proactively address any issues that arise, ensuring the AI models remain effective and reliable.
3. Governing AI-Ready Data in Context
Ongoing governance ensures that AI-ready data adheres to relevant policies and standards, supporting ethical and compliant AI use. Data stewardship involves enforcing policies throughout the data lifecycle, including observability metrics and model access controls. Proper stewardship guarantees that data management practices align with organizational goals and regulatory requirements.
Compliance with data and AI standards and regulations is non-negotiable. Emerging AI standards, such as the AI EU Act and existing regulations like GDPR, impose specific requirements on data management practices. Adhering to these standards ensures that AI applications remain legally compliant and ethically sound.
AI ethics requirements form a crucial part of governance. Ethical considerations, such as the acceptability of using real customer data for training models, must be addressed to prevent misuse and ensure fair treatment of individuals. Controlled inference and derivation are also important. Tracking and governing the use of AI model outputs, especially in composite AI systems, helps maintain transparency and accountability.
Data bias and fairness are specific challenges in AI. Bias in training data can lead to unfair and discriminatory outcomes. Governing data to anticipate and mitigate bias proactively ensures that AI models produce fair and unbiased results. Finally, data sharing supports delivering AI-ready data across various use cases. Facilitating data and metadata sharing promotes reuse and accelerates the development of AI-ready practices. Organizations can leverage a broader range of data sources and enhance their AI capabilities by enabling data sharing.
Conclusion
Preparing data for AI is a dynamic and iterative process that extends beyond traditional data management principles. For D&A leaders, understanding the specific requirements of AI-ready data is essential for leveraging AI effectively. Organizations can ensure their data is truly AI-ready by aligning data with use-case requirements, qualifying its use to meet confidence standards, and governing it within the context of AI applications. Embracing these practices will pave the way for successful AI implementations that drive innovation and deliver tangible business value.